
- 参会报名
- 会议介绍
- 会议日程
- 会议嘉宾
- 参会指南
-
手机下单
![]() |
![]() O'Reilly和Intel人工智能2019北京大会 已截止报名会议时间: 2019-06-18 08:00至 2019-06-21 18:00结束 会议地点: 北京 北京国际饭店会议中心 东城区建国门内大街9号 周边酒店预订
|
会议介绍
会议内容 往届会议 主办方介绍
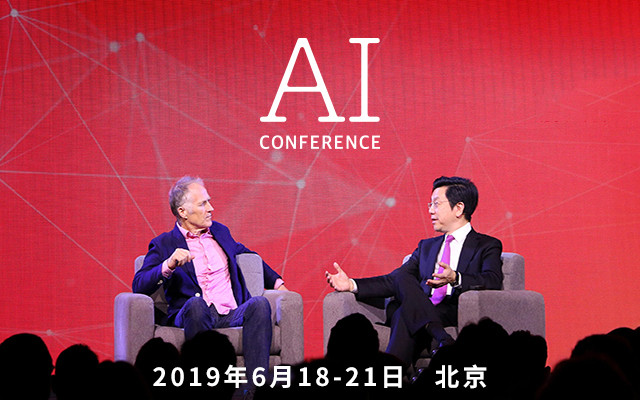
O'Reilly和Intel人工智能2019北京大会宣传图
人工智能大会把硅谷带到中国。
人工智能大会是无与伦比的世界领先创新者盛会,快来参与,寻找应用人工智能在商业上的各种机遇。
极度聚焦于技术内容和商业应用的交融,人工智能北京大会吸引了世界各地的顶尖人工智能人士。大会有4天信息满满的内容,包括实用性的分会场议题,深度培训课程,极具启发性的主题演讲,以及难得的思想交流与碰撞的社交机会。
极具启发性的主题演讲、2天的培训课程、执行简报、案例研究、半天的辅导课和深度实践性的议题会带来数据领域最新及最重要的内容。
人工智能大会:将人工智能在工作中用起来
本次大会的独特之处在于将重点放在应用人工智能——弥合人工智能研究领域与产业商业应用之间的差距。
只有本次北京人工智能大会才将硅谷和中国融合在一起,创造一次全球人工智能专家难得的相聚,并为日程安排带来非同寻常的技术深度及实用的业务内容。讲师为来自各公司人工智能专家,包括百度、谷歌、eBay、Bonsai、Uber、微软、阿里巴巴、亚马逊、SAS、Unity、SalesForce、IBM、伯克利、斯坦福及牛津大学——仅为部分公司。
无论你的关注点在哪里都将在本次人工智能大会上找到:
企业中的人工智能:执行简报,案例研究及用例,行业特定应用
人工智能对商业及社会的影响:自动化,安全,规范
实施人工智能项目:应用,工具,架构,安全
与人工智能交互:设计,指标,产品管理,机器人
模型及方法:增强及机器学习,TensorFlow,深度学习,GAN,自然语言处理及理解,语音识别,计算机视觉
我们一起将人工智能在工作中用起来
人工智能培训课程
将自己沉浸在两天针对关键主题的课程中。培训课程安排在6月18-19日进行,控制班级规模以保证最好的学习体验(包括与讲师互动)。
量化互联网金融信用与反欺诈风控
Deep Learning with PyTorch
Deep Learning with TensorFlow
Professional Kafka development
人工智能大会的多样性和包容性
我们相信真正的创新依赖于听取有着多样化视角的人们的观点。
为了创造最好的未来,我们需要每个人
在O'Reilly,我们相信真正的创新取决于对具有各种观点的人的倾听和聆听。我们希望我们的学习平台,我们的会议(以及参与其中的技术社区和公司)以及整个组织包括,鼓励和认可所有种族,民族,性别,年龄,能力,宗教,性取向的人,和兵役。我们还没有。
我们承诺对于参加我们任何活动的每个人给予一个安全、富有成效的环境。
在O'Reilly,我们假设大多数人都是聪明且善意的,我们并不倾向于告诉人们该做什么。但是,我们希望每个O'Reilly会议都能成为一个安全且富有成效的环境。为此,本行为准则阐明了我们支持的行为,并不支持会议。我们方法的核心是:
我们不会宽恕在会议场所或任何地方的骚扰或冒犯行为。这与我们公司的价值观背道而驰。更重要的是,这与我们作为人类的价值观背道而驰。
查看更多
人工智能(AI)终于从大学实验室的圈子走出而成为软件开发人员工具集的重要组成部分,也成为主流公司的关注点。 相对于学术会议上展示的研究成果,业界对于应用人工智能的信息有着急剧增长且紧迫的需求。
所以我们组织、创办了这个人工智能大会。
人工智能大会是最前沿科学研究与商业实现交融与碰撞的地方。它是一个供大家深入探讨人工智能技巧和技术的殿堂,特别专注于人工智能技术如何应用于真实世界中的应用。在这里你将可以仔细剖析案例,深入钻研最新研究成果,学习如何在自己项目中实现人工智能,分享在智能工程和应用中正在出现的最佳实践,揭示人工智能的局限及未被发掘的机遇,并参与讨论人工智能将会如何改变商业世界的版图。
2017年6月29日O'Reilly与Intel Nervana宣布建立合作伙伴关系,共同举办人工智能大会。
探索在业务中应用人工智能的机会
本次人工智能大会将硅谷带到中国
全球两大人工智能中心相聚在一起——本次人工智能北京大会是世界范围内领先的应用人工智能创新者史无前例的大聚会
多数会议议题为中文议题,部分有选择的议题采用英文。
人工智能大会:将人工智能在工作中用起来
本次大会的独特之处在于将重点放在应用人工智能——弥合人工智能研究领域与产业商业应用之间的差距。
只有本次北京人工智能大会才将硅谷和中国融合在一起,创造一次全球人工智能专家难得的相聚,并为日程安排带来非同寻常的技术深度及实用的业务内容。讲师为来自各公司人工智能专家,包括百度、谷歌、eBay、Bonsai、Uber、微软、阿里巴巴、亚马逊、SAS、Unity、SalesForce、IBM、伯克利、斯坦福及牛津大学——仅为部分公司。
无论你的关注点在哪里都将在本次人工智能大会上找到:
企业中的人工智能:执行简报,案例研究及用例,行业特定应用
人工智能对商业及社会的影响:自动化,安全,规范
实施人工智能项目:应用,工具,架构,安全
与人工智能交互:设计,指标,产品管理,机器人
模型及方法:增强及机器学习,TensorFlow,深度学习,GAN,自然语言处理及理解,语音识别,计算机视觉
继在纽约和旧金山成功举办(均售罄)后人工智能大会于2018年4月10-13日莅临北京。欢迎加入,学习如何在工作中应用人工智能。
为什么应该参加
如果你希望理解人工智能将会如何影响商业世界的版图,或是正在参与深度学习或人工智能的工作(或计划参与)—那你应该参加本次人工智能大会,你将能够:
成为首批先锋,理解如何利用这一巨变以及如何在颠覆性结局下生存。
找到跨行业跨领域利用已有人工智能资源的新方法。
学习如何将人工智能从学术项目引入到真正的商业应用。
发现学习、招聘和就业的机会。
与其他创新人士和思想领袖面对面交流。
你会遇到谁
技术人员
算法工程师、算法科学家
CxO(x可以是分析、数据、信息、创新或技术)
数据科学家、数据工程师
研究科学家
软件工程师
商业与战略人士
业务分析师
业务经理、战略制定人士和决策人士
首席创新官、创新经理、创新主管
首席投资官/经理
产品市场推广经理、产品经理和项目经理
人工智能大会体验
人工智能大会是那些应用人工智能领域最聪明大脑聚集在一起的独特机遇,共同经历日程密集、令人兴奋的四天时间。你将会发现:
极具启发性的主题演讲与实用且信息量丰富的议题,探索最新人工智能进展、案例研究和最佳实践
教学辅导课与培训课程,专门设计来深度考察工具、算法和应用
与迅速发展的人工智能领域中数百名工程师、研究人员、开发人员、项目与产品经理、创新主管以及其他深度学习专家建立关系的好机会
一个明亮的“大厅走廊”方便与会者、讲师和记者就重要话题进行辩论与讨论
查看更多
会议日程
(最终日程以会议现场为准)
周二&周三 周三 周四 周五 培训课程
Deep Learning with PyTorch
多功能厅6A+B (Function Room 6A+B)
Rich Ott (The Data Incubator)
PyTorch is a machine learning library for Python that allows users to build deep neural networks with great flexibility. Its easy to use API and seamless use of GPUs make it a sought after tool for deep learning. This course will introduce the PyTorch workflow and demonstrate how to use it. Students will be equipped with the knowledge to build deep learning models using real-world datasets.
Deep Learning with TensorFlow
多功能厅5A+B(Function Room 5A+B)
与人工智能交互 (Interacting with AI)
Season Yang (McKinsey & Company)
The TensorFlow library provides for the use of computational graphs, with automatic parallelization across resources. This architecture is ideal for implementing neural networks. This training will introduce TensorFlow's capabilities in Python. It will move from building machine learning algorithms piece by piece to using the Keras API provided by TensorFlow with several hands-on applications.
量化互联网金融信用与反欺诈风控
多功能厅3A+B(Function Room 3A+B)
实施人工智能 (Implementing AI) , 模型与方法 (Models & Methods)
黄铃 (Tsinghua University)
陈薇 (排列科技)
您想了解金融企业是怎样利用大数据和人工智能技术来画像个人行为并检测欺诈用户的吗?互联网金融幕后的量化分析流程是怎么杨的?个人信用是怎样通过大数据被量化的?在实践过程中,机器学习算法的应用存在着哪些需要关注的方面?怎样通过图谱分析来融合多维数据,为我们区分正常用户和欺诈用户? 这套辅导课基于清华大学交叉信息研究院开设的一门"量化金融信用与风控分析”研究生课。其中会用LendingClub的真实借贷数据做为案例,解说一些具体模型的实现。
Professional Kafka development
多功能厅2(Function Room 2)
Jesse Anderson (Big Data Institute)
Jesse Anderson leads a deep dive into Apache Kafka. You'll learn how Kafka works and how to create real-time systems with it. You'll also discover how to create consumers and publishers in Kafka and how to use Kafka Streams, Kafka Connect, and KSQL as you explore the Kafka ecosystem.
查看更多
A practical guide towards explainability and bias evaluation in machine learning
紫金大厅B(Grand Hall B)
与人工智能交互 (Interacting with AI) , 实施人工智能 (Implementing AI) , 模型与方法 (Models & Methods)
Alejandro Saucedo (The Institute for Ethical Ai & Machine Learning)
Undesired bias in machine learning has become a worrying topic due to the numerous high profile incidents. In this talk we demystify machine learning bias through a hands-on example. We'll be tasked to automate the loan approval process for a company, and introduce key tools and techniques from latest research that allow us to assess and mitigate undesired bias in our machine learning models.
Design Thinking for AI
紫金大厅B(Grand Hall B)
人工智能对商业及社会的影响 (Impact of AI on Business & Society)
Chris Butler (Philosophie)
Purpose, a well-defined problem, and trust from people are important factors to any system, especially those that employ AI. Chris Butler leads you through exercises that borrow from the principles of design thinking to help you create more impactful solutions and better team alignment.
基于深度学习的时间序列预测 (Deep Learning for Time Series Forecasting)
报告厅(Auditorium)
模型与方法 (Models & Methods)
Yijing Chen (Microsoft)
Dmitry Pechyoni (Microsoft)
Angus Taylor (Microsoft)
Vanja Paunic (Microsoft)
Henry Zeng (Microsoft)
Almost every business today uses forecasting to make better decisions and allocate resources more effectively. Deep learning has achieved a lot of success in computer vision, text and speech processing, but has only recently been applied to time series forecasting. In this tutorial we show how and when to apply deep neural networks to time series forecasting. The tutorial will be in CHN and EN.
通过自动化机器学习民主化和加速AI落地 (Democratizing and Accelerating AI through Automated Machine Learning)
报告厅(Auditorium)
实施人工智能 (Implementing AI) , 模型与方法 (Models & Methods)
Sujatha Sagiraju (Microsoft), Henry Zeng (Microsoft)
Intelligent experiences powered by AI can seem like magic to users. Developing them, however, is pretty cumbersome involving a series of sequential and interconnected decisions along the way that are pretty time consuming. What if there was an automated service that identifies the best machine learning pipelines for a given problem/data? Automated machine learning does exactly that!
Analytics Zoo: Distributed Tensorflow and Keras on Apache Spark
多功能厅8A+B(Function Room 8A+B)
实施人工智能 (Implementing AI)
Zhichao Li (Intel)
In this tutorial, we will show how to build and productionize deep learning applications for Big Data using "Analytics Zoo":https://github.com/intel-analytics/analytics-zoo (a unified analytics + AI platform that seamlessly unites Spark, TensorFlow, Keras and BigDL programs into an integrated pipeline) using real-world use cases (such as JD.com, MLSListings, World Bank, Baosight, Midea/KUKA, etc.)
Building reinforcement learning models and AI applications with Ray
多功能厅8A+B(Function Room 8A+B)
英文讲话 (Presented in English)
Richard Liaw (UC Berkeley RISELab)
Ray is a general purpose framework for programming your cluster. We will lead a deep dive into Ray, walking you through its API and system architecture and sharing application examples, including several state-of-the-art AI algorithms.
查看更多
Session
多功能厅2(Function Room 2)
Session
紫金大厅B(Grand Hall B)
Session
紫金大厅B(Grand Hall B)
Using deep learning and time-series forecasting to reduce transit delays
紫金大厅B(Grand Hall B)
与人工智能交互 (Interacting with AI) , 实施人工智能 (Implementing AI) , 英文讲话 (Presented in English)
Mark Ryan (IBM),
Alina Li Zhang (Nobul)
Toronto is unique among North American cities for having a legacy streetcar network as an integral part of its transit system. This means streetcar delays are a major contributor to gridlock in the city. Using deep learning and time-series forecasting, we'll show how streetcar delays can be predicted... and prevented.
Hacking Humans Made Easy: Signal Processing + AI + Video
紫金大厅B(Grand Hall B)
人工智能对商业及社会的影响 (Impact of AI on Business & Society) , 英文讲话 (Presented in English)
David Maman (Binah.ai)
Zero-day attacks. IoT-based botnets. Cybercriminal AI v. cyberdefender AI. While these won’t be going away, they aren’t the biggest worry we have in cybercrime. Hacking humans is. The combination of mere minutes of video, signal processing, remote heart rate monitoring, AI, machine learning, and data science can identify a person’s health vulnerabilities, which evildoers can make worse.
AI技术在外卖个性化场景中的落地与思考
报告厅(Auditorium)
实施人工智能 (Implementing AI)
刘先生 (美团)
该议题的内容包括: 1.外卖个性化场景:个性化搜索,个性化推荐 2.个性化产品形态包括:商家、商品、套餐等 3.外卖个性化中应用的AI技术包括:NLP,DNN,图像技术,强化学习 4.针对外卖业务的特点,介绍个性化场景中,几项重点AI技术的落地、挑战与思考
ONNX:开放和互操作平台让AI无处不在(AI everywhere: Open and interoperable platform for AI with ONNX)
报告厅(Auditorium)
实施人工智能 (Implementing AI) , 模型与方法 (Models & Methods)
Prasanth Pulavarthi (Microsoft), Henry Zeng (Microsoft)
An open and interoperable ecosystem enables you to choose the framework that's right for you, train at scale, and deploy to cloud and edge. ONNX provides a common format supported by many popular frameworks and hardware accelerators. This session provides an introduction to ONNX and its core concepts. The session will be delivered in English and Chinese jointly.
Exciting new features in TensorFlow 2.0
报告厅(Auditorium)
实施人工智能 (Implementing AI)
Tiezhen Wang (Google)
TensorFlow 2.0 is a major milestone with a focus on ease of use. This talk will give a in depth introduction to the new exciting features and best practices. Topics such as distributed strategies and edge deployment (TensorFlow Lite and TensorFlow.js) will also be covered.
自动机器学习(automated machine learning)技术的实践与应用
报告厅(Auditorium)
实施人工智能 (Implementing AI) , 模型与方法 (Models & Methods)
Hui Xue (微软亚洲研究院)
人工智能在过去的几年里飞速发展,但是机器学习的实践和应用需要消耗一定的人力和时间。例如,如何去做特征选择,如何设计一个适合该任务的神经网络模型等等。而自动机器学习技术,可以帮助开发者和机器学习实战者,缩短开发周期,提高效率。我们的介绍主要包括:自动机器学习技术的进展;我们开源的自动机器学习开源库Neural Network Intelligence; 如何利用自动机器学习的技术,在产品和应用上提高效率,节省所需的时间和缩短周期。我们会在最后一部分,分享一些利用自动特征选择,自动参数调整以及模型架构搜索上的成功案例。
打造A.I.闭环 引领产业变革
报告厅(Auditorium)
人工智能对商业及社会的影响 (Impact of AI on Business & Society)
温浩 (云从科技)
AI企业发展应该是一个从学术研究、行业验证、商业落地、行业平台到智能生态的一层层深入过程,这也是人工智能企业理想的发展阶段。 云从科技计划打造核心技术闭环,让计算机更好地服务人类。并将全面降低人工智能准入门槛,让“AI普惠”成为可能。
The Unreasonable Effectiveness of Transfer Learning on NLP
多功能厅2(Function Room 2)
模型与方法 (Models & Methods) , 英文讲话 (Presented in English)
David Low (Pand.ai)
Transfer Learning has been proven to be a tremendous success in the Computer Vision field as a result of ImageNet competition. In the past months, the Natural Language Processing field has witnessed several breakthroughs with transfer learning, namely ELMo, Transformer, ULMFit and BERT. In this talk, David will be showcasing the use of transfer learning on NLP application with SOTA accuracy.
The future of machine learning is decentralized
多功能厅2(Function Room 2)
英文讲话 (Presented in English) , 隐私、道德与规范 (Privacy, Ethics, & Compliance)
Alex Ingerman (Google)
Federated Learning is the approach of training ML models across a fleet of participating devices, without collecting their data in a central location. Alex Ingerman introduces Federated Learning, compares the traditional and federated ML workflows, and explores the current and upcoming use cases for decentralized machine learning, with examples from Google's deployment of this technology.
Session
多功能厅2(Function Room 2)
Efficient Deep Learning for the Edge
紫金大厅B(Grand Hall B)
模型与方法 (Models & Methods) , 英文讲话 (Presented in English)
Bichen Wu (UC Berkeley)
The success of deep neural networks is attributed to three factors: stronger computing capacity, more complex neural networks, and more data. These factors, however, are usually not available with the edge applications as autonomous driving, AR/VR, IoT, and so on. In this talk we discuss how we apply AutoML, SW/HW codesign, domain adaptation to solve these problems.
Session
多功能厅2(Function Room 2)
Session
多功能厅5A+B(Function Room 5A+B)
A Humane AI Solution to Improve Debt Collection
多功能厅5A+B(Function Room 5A+B)
实施人工智能 (Implementing AI)
Ying Liu (Abakus 鲸算科技(Wecash闪银))
AI debt collection platform of Abakus provides a friendly and humane product solution which is designed for people who work in the live agents of the organization in the frontline. The agent training of the organization could be enhanced more smoothly with an AI friendly culture. It has been proved in our experiment that the performance of the collection assistants has been highly improved.
线上财富管理领域中的AI应用
多功能厅5A+B(Function Room 5A+B)
执行简报/最佳实践 (Executive Briefing/Best Practices)
杨博理 (宜信大数据创新中心)
AI技术是线上财富管理领域中不可或缺的一环。在这个演讲中,我会将财富管理进一步细分为投资和实现财务目标两个方面,并分别讲解AI技术在这两个细分层面上的应用问题。对于投资而言,一些具备强金融逻辑的变量可能更适合使用机器学习进行预测。而在资产价格的预测上,可以尝试使用AI和大数据技术获取更多的有价值信息。对于实现财务目标而言,基于NLP技术的语义理解、引导式对话是理解用户的关键,基于AI和大数据的KYC也是判断用户状态的有效工具,而一个融合了财务规划、投资和精算知识的专家系统则是定制级规划的核心。
Real-time product recommendations leveraging deep learning on Apache Spark in Office Depot
多功能厅5A+B(Function Room 5A+B)
实施人工智能 (Implementing AI) , 模型与方法 (Models & Methods)
Guoqiong Song (Intel)
Luyang Wang (Office Depot)
Jennie Wang (Intel)
Jing (Nicole) Kong (Office Depot)
To show case how to build efficient recommender systems for e-commerce industry using deep learning technologies
Session
多功能厅5A+B(Function Room 5A+B)
Session
多功能厅6A+B (Function Room 6A+B)
Forecasting Customer Activities with Dilated Convolution Neural Networks: Use Case and Best Practices
多功能厅6A+B (Function Room 6A+B)
模型与方法 (Models & Methods)
Tao Lu (Microsoft)
Chenhui Hu (Microsoft)
Forecasting customer activities is one of the most important and common business problems. In Microsoft Azure Identity team, we forecast customer behavior based on billions of user activities. We will share how we improve 25% of forecasting accuracy with dilated convolutional neural networks and reduce 80% of the time in development with the best practices of time series forecasting.
Confidence Estimation for Deep Neural Networks
多功能厅6A+B (Function Room 6A+B)
模型与方法 (Models & Methods)
Jialin Jiao (Pony.ai)
While deep learning has been in the center of AI with unprecedentedly great results, predictions of deep neural networks usually do not come with a reliable and well-calibrated confidence score. Wrong but confident predictions place great threads to critical real-life applications, e.g. self-driving car. This talk is a tutorial/comparison of confidence estimation methods for deep neural networks.
Session
多功能厅6A+B (Function Room 6A+B)
Session
多功能厅6A+B (Function Room 6A+B)
快速社交 (Speed Networking)
本次人工智能会议上午8:00-8:30可以和希望社交的与会来宾见面。我们将在周五主题演讲之前搞一个非正式快速社交活动。一定记得带名片参加活动。
查看更多
Best practice of building data science platform in Rakuten
多功能厅2(Function Room 2)
与人工智能交互 (Interacting with AI) , 实施人工智能 (Implementing AI)
安敖日奇朗 (Rakuten, Inc.)
TzuLin Chin (Rakuten, Inc.)
Data Science Platform is a suite of tools for exploring data, training models, and running GPU/CPU compute jobs in an isolated container environment. It provides one click machine learning environment creation, powerful job scheduler and flexible "function as a service" component. It runs on Kubernetes and supports both on-premises and cloud environment, as well as hybrid mode.
Session with Joe Spisak
紫金大厅B(Grand Hall B)
Joseph Spisak (Facebook)
Session with Joe Spisak
Session
紫金大厅B(Grand Hall B)
ML Ops and Kubeflow Pipeline
紫金大厅B(Grand Hall B)
实施人工智能 (Implementing AI) , 模型与方法 (Models & Methods)
Kazunori Sato (Google)
Creating an ML model is just a starting point. To bring the technology into production service, you need to solve various real-world issues such as: building a data pipeline for continuous training, automated validation of the model, version control of the model, scalable serving infra, and ongoing operation of the ML infra with monitoring and alerting.
AI at ING: the why, how, and what of a data-driven enterprise
紫金大厅B(Grand Hall B)
执行简报/最佳实践 (Executive Briefing/Best Practices) , 文化与组织 (Culture & Organization) , 案例研究 (Case Studies)
Bas Geerdink (ING)
AI is at the core of ING’s business. We are a data-driven enterprise, with ‘analytics skills’ as a top strategic priority. We are investing in AI, big data, and analytics to improve business processes such as balance forecasting, fraud detection and customer relation management. In this talk, Bas will give an overview of the use cases and technology to inspire the audience!
Analytics Zoo: Distributed TensorFlow in Production on Apache Spark
报告厅(Auditorium)
实施人工智能 (Implementing AI)
Yang Wang (Intel)
We will introduce Analytics Zoo, a unified analytics + AI platform for distributed TensorFlow, Keras and BigDL on Apache Spark, designed for production environment. It enables easy deployment, high performance and efficient model serving for deep learning applications.
Sparkling: 基于Apache Spark进行一站式机器学习
报告厅(Auditorium)
实施人工智能 (Implementing AI)
Yiheng Wang (Tencent)
机器学习项目在企业中实际落地往往涉及到复杂工作流构建和数据管理,以及多种工具的整合。而且随着数据规模的增加,团队规模的扩大,这一任务更具挑战性。Apache Spark是业界流行的大数据框架,被广泛的应用在海量数据的分析处理。本议题将介绍我们在腾讯云上如何基于Apache Spark为客户建立一个一站式机器学习平台的相关工作。主要内容包括多种数据源的接入,构建复杂数据管线,利用数据可视化理解数据,通过可插拔的机制使用各种流行的机器学习框架,以及部署和监控模型。我们也会分享在这一过程中遇到的问题和挑战。听众也可以了解到,通过这种和大数据紧密结合的一站式机器学习,用户可以怎样更加高效的建立和管理他们的机器学习项目,从而加速了机器学习在业务中的落地。
AVA: a Cloud-Native Deep Learning Platform at Qiniu
报告厅(Auditorium)
实施人工智能 (Implementing AI)
Chaoguang Li (Qiniu)
Bin Fan (Alluxio)
Atlab Lab at Qiniu Cloud focuses on deep learning for computer vision. Our team has built a high-performance and cost-effective training platform based on Cloud for deep learning, called AVA, which deeply integrates open source software stack including Tensorflow, Caffe, Alluxio and KODO our own cloud object storage.
Query the planet: Geospatial big data analytics at Uber
报告厅(Auditorium)
实施人工智能 (Implementing AI)
Zhenxiao Luo (Uber)
One of the distinct challenges for Uber is analyzing geospatial big data. Locations and trips provide insights that can improve business decisions and better serve users. Geospatial data analysis is particularly challenging, especially in a big data scenario. For these analytical requests, we must achieve efficiency, usability, and scalability in order to meet user needs and business requirements.
保险中的机器学习实践
报告厅(Auditorium)
实施人工智能 (Implementing AI)
鞠芳 (中国人寿研发中心)
分析保险行业人工智能发展情况及现有数据特性,评估机器学习模型构建的主流工具、语言、算法。总结基于机器学习技术,实现一个保险业人工智能场景的全流程——从场景研讨、数据加工提取到模型构建、模型效果评估、模型落地实施。以一个真实的机器学习模型项目为例,介绍整个方法论不同环节中各方人员的参与工作内容和比例,探讨特征稳定性、样本不均衡、参数选择、模型可解释性等环节的难点及尝试方案。为金融或者其他行业的机器学习项目落地提供参考和指导。
Achieving Salesforce-Scale Machine Learning in Production
多功能厅2(Function Room 2)
执行简报/最佳实践 (Executive Briefing/Best Practices)
Sarah Aerni (Salesforce Einstein)
At Salesforce Einstein data science is an agile partner to over 100,000 customers. How do we achieve this scale? We share lessons learned in business, technology and process along the way. Via use cases, oft-missed foundational elements for deployment, and the evaluations that must happen along the way, we will share how to achieve and sustain models in production, and where to go from there.
AI in the Newsroom: Helping Authors Do What They Do Best
多功能厅2(Function Room 2)
与人工智能交互 (Interacting with AI)
Boris Yakubchik (Forbes)
Forbes has been working on a custom publishing platform and we have integrated machine learning into it from the ground up. We provide an assistant to our writers that maintains a live connection and helps them as they work. In this talk we’ll share our system design, discuss choices we had to make along the way, and walk through several examples.
Session
多功能厅2(Function Room 2)
Session
紫金大厅B(Grand Hall B)
Using ML for personalizing Food Recommendations
多功能厅2(Function Room 2)
模型与方法 (Models & Methods)
Maulik Soneji (Go-jek)
Jewel James (Go-jek)
The story of how we prototyped the search framework that personalizes the restaurant search results by using ML to learn what constitutes a relevant restaurant given a user's purchasing history
自动驾驶技术是如何应用于新潮传媒、新零售行业
多功能厅5A+B(Function Room 5A+B)
实施人工智能 (Implementing AI)
Li Yuan (Perceptin 深圳普思英察科技有限公司)
如何令自动驾驶技术落地并结合新潮传媒以及新零售业务,相关的技术是如何实现,商业模式是什么以及如何通过人工只能技术提升行业的效率。
How China Telecom combats financial frauds with Adversarial AutoEncoder?
多功能厅5A+B(Function Room 5A+B)
案例研究 (Case Studies)
Weisheng Xie (China Telecom BestPay Co., Ltd)
We exploit the good representation capability of AAE (Adversarial AutoEncoder) in our risk factors modeling in fighting a special kind of financial frauds. It's one step of our long stack of unsupervised tasks, yet it's proved to be efficient and effective in our practice.
人工智能病理影像辅助诊断系统——从方法到落地
多功能厅5A+B(Function Room 5A+B)
与人工智能交互 (Interacting with AI) , 实施人工智能 (Implementing AI)
王书浩 (透彻影像)
病理学是医学诊断的“金标准”,病理报告对于临床医生提供进一步治疗策略至关重要。一位能够独立发病理报告的病理医师需要10年以上的培养周期,我国目前共有约1万名注册在案的病理医师,根据WHO的要求,人才缺口为4-9万人。使用人工智能来辅助病理医师对样本进行诊断,不仅能够大幅提高医师的诊断效率,而且可以减少漏诊,提高诊断准确率。数字化的病理影像能够观察到组织的细胞形态,在最高倍数字扫描时,文件尺寸达到GB量级,需要从人工智能和系统工程的层面去应对这些挑战。在这个演讲中,我们将从人工智能系统的构建方法入手,介绍透彻影像与中国人民解放军总医院在消化道病理影像辅助系统研发过程中的技术细节。同时,我们将分享诊断系统从部署到落地使用的一些经验。
Session
多功能厅5A+B(Function Room 5A+B)
基于目标检测的智能化成矿异常信息提取
多功能厅5A+B(Function Room 5A+B)
实施人工智能 (Implementing AI)
李苍柏 (中国地质科学院矿产资源研究所)
矿床所在的位置往往伴随着地质、地球物理、地球化学、遥感异常,因此,这些异常所在的位置也往往伴随着矿床的存在。所以,在找矿工作当中,一个重要的过程便是在地、物、化、遥数据中寻找异常,并将其整合,得出该区域成矿的概率,从而推断出靶区所在的位置。但传统方法并未考虑空间中点与点之间的相关关系。而卷积神经网络中的卷积和池化方法,充分考虑了点与点之间的相关关系。但单纯使用卷积神经网络只能进行特征提取,不能圈定异常所在的区域。因此,特将目标检测的相关算法引入其中,从而圈定异常所在的区域。
Session
多功能厅6A+B (Function Room 6A+B)
在边缘实现深度学习
多功能厅6A+B (Function Room 6A+B)
模型与方法 (Models & Methods)
陈玉荣 (Intel)
深度学习在许多领域尤其是视觉识别/理解方面取得了巨大突破,但它在训练和部署方面都存在一些挑战。本讲座将介绍我们通过高效CNN算法设计、领先DNN模型压缩技术和创新部署时DNN网络结构优化来解决深度学习部署挑战的前沿研究成果。
非监督学习在大规模图谱上的案例应用和开源算法剖析
多功能厅6A+B (Function Room 6A+B)
模型与方法 (Models & Methods)
Mingxi Wu (TigerGraph)
图数据上的非监督学习在激活大数据的经济价值上有着广泛和不可替代的作用。 PageRank能够发掘重要的实体, 社区发掘(community detection)可以找到具有某种特性的群体,紧密度中心性算法(Closeness Centrality)可以自动找到远离群体的个体。所有这些算法都是非监督的学习。 我们分享一些具体客户案例来展示他们的价值,同时分享怎样在大数据上灵活应用这些开源算法。
PAI Tensor Accelerator and Optimizer---Yet Another Deep Learning Compiler
多功能厅6A+B (Function Room 6A+B)
与人工智能交互 (Interacting with AI) , 实施人工智能 (Implementing AI)
杨军 (阿里巴巴)
龙国平 (Alibaba)
本次演讲会介绍阿里计算平台PAI团队过去一年多时间里在深度学习编译器领域的技术工作进展----PAI TAO(Tensor Accelerator and Optimizer)。PAI-TAO采用通用编译优化技术,来解决PAI平台所承载的多样性AI workload面临的训练及推理需求的性能优化问题,在部分workload上获得了20%到4X不等的显著加速效果,并且基本作到用户层全透明,在显著提升平台效率性能的同时也有效照顾了用户的使用惯性。目前PAI-TAO已经先后用于支持阿里内部搜索、推荐、图像、文本等多个业务场景的日常训练及推理需求。
Low precision inference on Intel Architecture
多功能厅6A+B (Function Room 6A+B)
实施人工智能 (Implementing AI)
Lei Xia (Intel)
Vector Neural Network Instructions or VNNI is the new Intel instruction set for low precision AI inference inside next generation Xeon platform. This lecture is to introduce the features of the VNNI and Intel software tools to support developers to use this new instruction set to accelerate inference with INT8.
快速社交 (Speed Networking)
在本次人工智能大会上与寻求联系的与会者会面。会议将在周四主题演讲之前举行一个非正式的快速社交活动。一定要带上自己的名片来享受社交活动。
查看更多
所有培训课程安排在周二周三上午9:00至下午17:00。为了保证高水平的动手学习和与导师互动机会,全部培训课程都会限制在一定规模。 白金门票和培训门票不包含周三教学辅导课。
09:00 - 17:00 Tuesday, April 10 & Wednesday, April 11
用TensorFlow进行深度学习
Location: 多功能厅5A+B(Function Room 5A+B)
TensorFlow是一个流行的深度学习的工具。我们会介绍TensorFlow的流程图、学习使用它的Python API,并展示它的用处。我们会从简单的机器学习算法开始,然后实现神经网络。我们还会讨论一些真实的深度学习的应用,包括机器视觉、文本处理和生成型网络。
09:00 - 17:00 Tuesday, April 10 & Wednesday, April 11
用Deeplearning4j框架构建神经网络分析时间序列
Location: 多功能厅6A+B (Function Room 6A+B)
Secondary topics: 深度学习(Deep Learning), 金融服务 (Financial Services)
在分析时间序列或者序列数据方面循环神经网络(RNN)已经被证明是非常有效的,那么在实际的案例中如何才能把循环神经网络(RNN)的优点发挥出来呐?这里将演示如何用Deeplearning4j框架构建循环神经网络(RNN)来解决时间序列的问题。
09:00 - 17:00 Tuesday, April 10 & Wednesday, April 11
人工智能和金融科技:量化金融信用与欺诈风险的评估
Location: 多功能厅3A+B(Function Room 3A+B)
您想了解金融企业是怎样利用大数据和人工智能技术来画像个人行为并检测欺诈用户的吗?互联网金融幕后的量化分析流程是怎么杨的?个人信用是怎样通过大数据被量化的?在实践过程中,机器学习算法的应用存在着哪些需要关注的方面?怎样通过图谱分析来融合多维数据,为我们区分正常用户和欺诈用户? 这套辅导课基于清华大学交叉信息研究院2017年春天新开设的一门"量化金融信用与风控分析”研究生课。其中会用LendingClub的真实借贷数据做为案例,解说一些具体模型的实现。
查看更多
会议嘉宾
(最终出席嘉宾以会议现场为准)
查看更多
参会指南
会议门票 场馆介绍
白金门票 周二~周五 | 白银门票 周三~周五 | 青铜门票 周四~周五 | 培训门票 周二~周三 | |
¥5300(05.10前) ¥5700现在注册 原价¥6100 |
¥3200(05.10前) ¥3600现在注册 原价¥4000 |
¥2500(05.10前) ¥2900现在注册 原价¥3300 |
| |
一门两天培训课程(周二—周三; 不包含教学辅导课) | Y | Y | ||
所有教学辅导课 (周三) | Y | |||
所有主题演讲&议题 (周四—周五) | Y | Y | Y | |
赞助商区域&所有社交活动 | Y | Y | Y | Y |
90天O'Reilly online learning会员 | Y | Y | Y | Y |
请注意:
白银和青铜门票不包含周二及周三的培训课程。
白金、青铜和培训门票不包含周三的教学辅导课。
标准折扣不适用于白金门票及培训门票。
标准折扣:(可添加客服微信咨询:hdjzixun)
往届来宾折扣
如果您参加过以往任何一届AI Conference 大会,将享有八折折扣。
公司团队折扣
如果一个公司注册3-5人则享受八折。
如果你的公司计划派遣6人或者更多人参加AI Conference我们可以提供更大折扣:
6-9人:七五折
10人或10人以上:七折
政府折扣
政府机构全职雇员可以享受八折。
学术人士折扣
学术机构全职雇员可以享受八折。
学术导师折扣
全职学术导师享受五折门票,需要有机构信笺证明其学术状态。学术导师折扣数量有限。
全日制学生折扣
为全日制学生提供三五折折扣,必须提供证明:学生证复印件以及能表明每学期注册了12学分或更多学分的课时表复印件。全日制学生折扣数量有限。
非营利组织折扣
给予非营利组织全职雇员六折折扣,需要出示全职雇员证明以及非营利组织证明(501 c3或同等效力证明)。非营利组织折扣数量有限。
取消和转让政策:
如果您必须取消一定要在2019年5月20日之前书面通知我们。请提前告知工作人员。
会议开始前30天之内取消是不退款的。2019年6月3日之前您可以把注册转让给其他人,一定要将授权发送给工作人员。
确认并完成支付后取消的参会者、或者超过截止日期取消的参会者要承担全部会议费用。
遇到极端情况该会议取消O'Reilly Media, Inc.的责任仅限于退回支付的注册款项。
查看更多
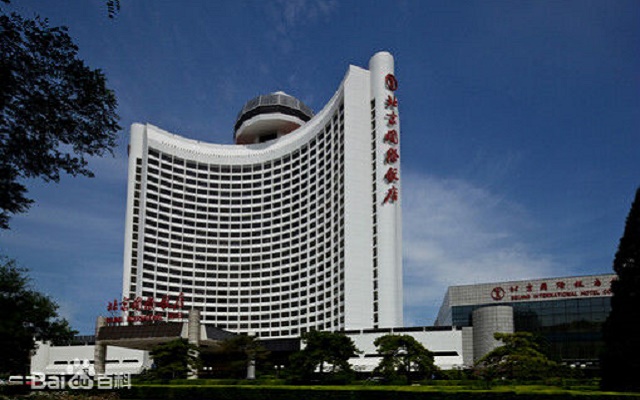
交通指南:
离机场距离(公里):24; 离北京火车站距离(公里):2; 离市中心距离(公里):2.5; 离建国门距离(公里):2;
五星级的商务酒店北京国际饭店,位于长安街上,面向恒基中心、中粮广场,距北京站仅咫迟之遥,酒店2002年由国外设计师重新设计全面装修,极具欧式风情,客房的设计古典而现代,顶层的旋转餐厅可俯视北京长安街上的浪漫夜景。酒店1987年12月开业,2002年重新装修,楼高29层,共有客房总数993间套。客房设有中央空调控制系统、先进的音响、闭路电视、迷你型酒吧、冰箱、电子门锁及国际直拨电话。饭店由二十九层主楼及辅助裙房楼宇组成。机场班车、北京西站专线车可直达饭店,尽享交通便利;加上饭店完善、齐全的餐厅和娱乐设施,让您耳目一新,物有所值。地处北京的中央商务区、首都的心脏地带 - 东长安街上,毗邻人民大会堂、外经贸部、北京市政府、中国海关等国家机关, 与各国驻华使馆和各跨国公司中国区办事处近在咫尺, 距离亚洲最大的商业建筑群王府井步行街仅一街之遥, 距离首都飞机场仅有30分钟车程,交通畅捷、旺中取静,为商务及旅游人士居停北京之理想下榻之所。 酒店1987年12月开业,2002年重新装修,楼高29层,共有客房总数993间套。主楼是一幢呈三叉曲面体的白色高层建筑,宽阔的门前广场,点缀着绿柏、水池和喷泉,地上、地下停车场可同时停放大小汽车300辆。主楼外侧有幽静舒适的室外庭院。经过全面装修改造后的国际饭店,明亮宽敞的大堂、环境幽雅的四季酒吧、特色浓郁的"大上海"和"福临门"餐厅、鸟瞰京城的28层"星光旋转餐厅"、异域风情的日本餐厅、设备先进的商务中心,齐全的娱乐设施和会议中心;以及专为海内外公司、商社装修的办公楼层,全新的房间、明亮的灯光、高质量的管理。
温馨提示
酒店与住宿:
为防止极端情况下活动延期或取消,建议“异地客户”与活动家客服确认参会信息后,再安排出行与住宿。
退款规则:
活动各项资源需提前采购,购票后不支持退款,可以换人参加。
您可能还会关注
-
AICon 全球人工智能开发与应用大会 2025·上海
2025-05-23 上海
-
2024PM产品力领航者大会
2025-05-16 上海
-
AiCon北京2025|全球人工智能与大模型应用峰会
2025-12-18 北京
-
AiCon深圳2025|全球人工智能与大模型应用峰会
2025-08-22 深圳
部分参会单位
- 国际商业机器(中国)投资有限公司
- 国际商业机器(中国)投资有限公司
- 上海中山医疗科技发展公司
- 上海交通大学
- 华为技术有限公司
- 北京筑梦园科技有限公司沈阳分公司
- 北京中体骏彩信息技术有限公司
- 广发银行股份有限公司信用卡中心
- 国际商业机器(中国)投资有限公司
- 国际商业机器(中国)投资有限公司
- 上海中山医疗科技发展公司
- 上海交通大学
- 华为技术有限公司
- 北京筑梦园科技有限公司沈阳分公司
- 北京中体骏彩信息技术有限公司
- 广发银行股份有限公司信用卡中心
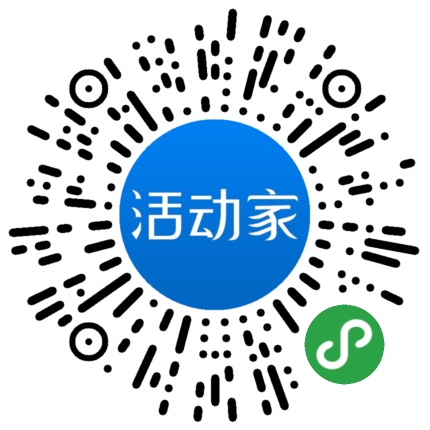
微信扫一扫
分享给朋友
邮件提醒通知